Algorithmic Fairness (AlFa)
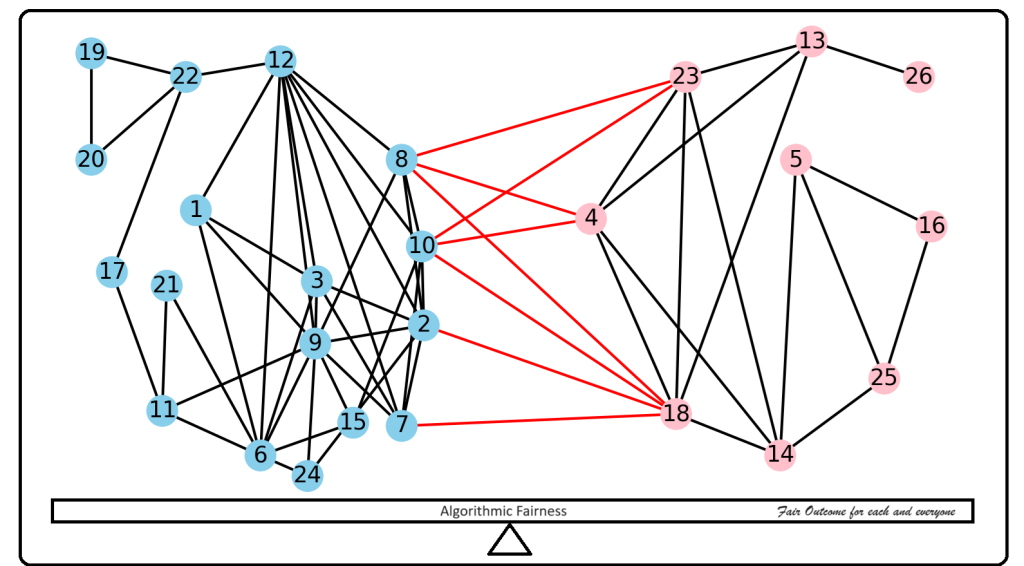
Algorithmic Fairness (AlFa) is a special interest group doing research on understanding biases and designing fair algorithms in Network Science and Data Science. This group is founded by Akrati Saxena and hosted at CNS, LIACS, the Department of AI and Computer Science of Leiden University. The group mainly focuses on the following four themes.
Themes
- Understanding Biases: This theme focuses on analyzing biases in complex social systems, such as understanding inequalities in complex systems, gender biases in communication systems, and biases in collaboration networks.
- Modeling Affirmative Actions: Once you understand existing biases, the focus is on designing interventions/affirmative actions to mitigate these biases. This mainly aims to the mathematical modeling of affirmative actions and Improving the visibility of minorities through these proposed interventions.
- Algorithmic Fairness: We focus on designing fair algorithms, defining fairness constraints and fairness evaluation metrics for network science and data science. The ongoing projects mainly focus on network structured datasets.
- Ethics in Data Science: Besides designing fair algorithms, it is also important to consider ethical data usage and design ethical policies for collecting data. Our group also focuses on algorithmic profiling, as it addresses critical ethical, social, and technical issues associated with the use of algorithms in various domains.
Group Members
Staff:
1. Dr. Akrati Saxena (Assistant Professor)
2. Fabrizio Corriera (PhD Candidate)
Students:
1. Wouter Burg (master student)
2. Fabian Jennrich (master student)
3. Amber van den Broek (master student)
4. Cesar Gesta (Bachelor student)
5. Christos Georghiou (Bachelor student)
6. Ashraf El Madkouki (Bachelor student)
7. Olof Tijs (Bachelor student)
8. Ray Sun (master student)
9. Daniel Gelencsér (master student)
Guest/Associated Members:
1. Vishal Sharma (PhD Candidate at IIT Roorkee, India)
2. Caroline Pena (Visiting PhD, 2024)
3. Stanisław Stępień (PhD Candidate at WUST, Poland)
Former members and affiliates:
1. Diego van Egmond (master student)
2. Elze de Vink (master student)
3. Bob J. van Beek (master student)
4. Seyidali Bulut (bachelor student)
5. Hanshu Yu MSc (researcher, 2023-2024)
Publications
- de Vink, E. and Saxena, A., 2025.Group Fairness Metrics for Community Detection Methods in Social Networks. Complex Networks Conference 2024
- de Groot, A., Fletcher, G., van Maanen, G., Saxena, A., Serebrenik, A. and Taylor, L., 2024. A canon is a blunt force instrument: data science, canons, and generative frictions. In Dialogues in Data Power (pp. 186-214). Bristol University Press.
- Saxena, A., Fletcher, G. and Pechenizkiy, M., 2024. Fairsna: Algorithmic fairness in social network analysis. ACM Computing Surveys.
- Fajri, R., Saxena, A., Pei, Y., and Pechenizkiy, M. FAL-CUR: Fair Active Learning using Uncertainty and Representativeness on Fair Clustering. Expert Systems With Applications Journal, 2023
- Saxena, A., Gutiérrez Bierbooms, C. and Pechenizkiy, M., 2023. Fairness-aware fake news mitigation using counter information propagation. Applied Intelligence, pp.1-22.
https://doi.org/10.1007/s10489-023-04928-3 - Liang, Z., Li, Y., Huang, T., Saxena, A., Pei, Y., and Pechenizkiy, M. Heterophily-Based Graph Neural Network for Imbalanced Classification. Complex Networks Conference 2023 (accepted)
- Saxena, A., Sethiya, N., Saini, J.S., Gupta, Y. and Iyengar, S.R.S., 2023, February. Social network analysis of the caste-based reservation system in India. In Computational Data and Social Networks: 11th International Conference, CSoNet 2022, Virtual Event, December 5–7, 2022, Proceedings (pp. 203-214). Cham: Springer Nature Switzerland.
https://doi.org/10.1007/978-3-031-26303-3_18 - Saxena, A., Fletcher, G. and Pechenizkiy, M., 2022. Nodesim: Node similarity based network embedding for diverse link prediction. EPJ Data Science, 11(1), pp.1-22.
https://doi.org/10.1140/epjds/s13688-022-00336-8 - Saxena, A., Fletcher, G., & Pechenizkiy, M. (2021). HM-EIICT: Fairness-aware link prediction in complex networks using community information. Journal of Combinatorial Optimization, 1-18.
https://doi.org/10.1007/s10878-021-00788-0 - Doewes, A., Saxena, A., Pei, Y., & Pechenizkiy, M., (2022) Individual Fairness Evaluation for Automated Essay Scoring System. Proceedings of the 15th International Conference on Educational Data Mining, 206–216. https://doi.org/10.5281/zenodo.6853150
- Saxena, A., Fletcher, G., & Pechenizkiy, M. (2021, April). How Fair is Fairness-aware Representative Ranking?. In Companion Proceedings of the Web Conference 2021 (pp. 161-165).
https://doi.org/10.1145/3442442.3453458